The Impact of Semantic Segmentation Labeling Tools on Business and Software Development
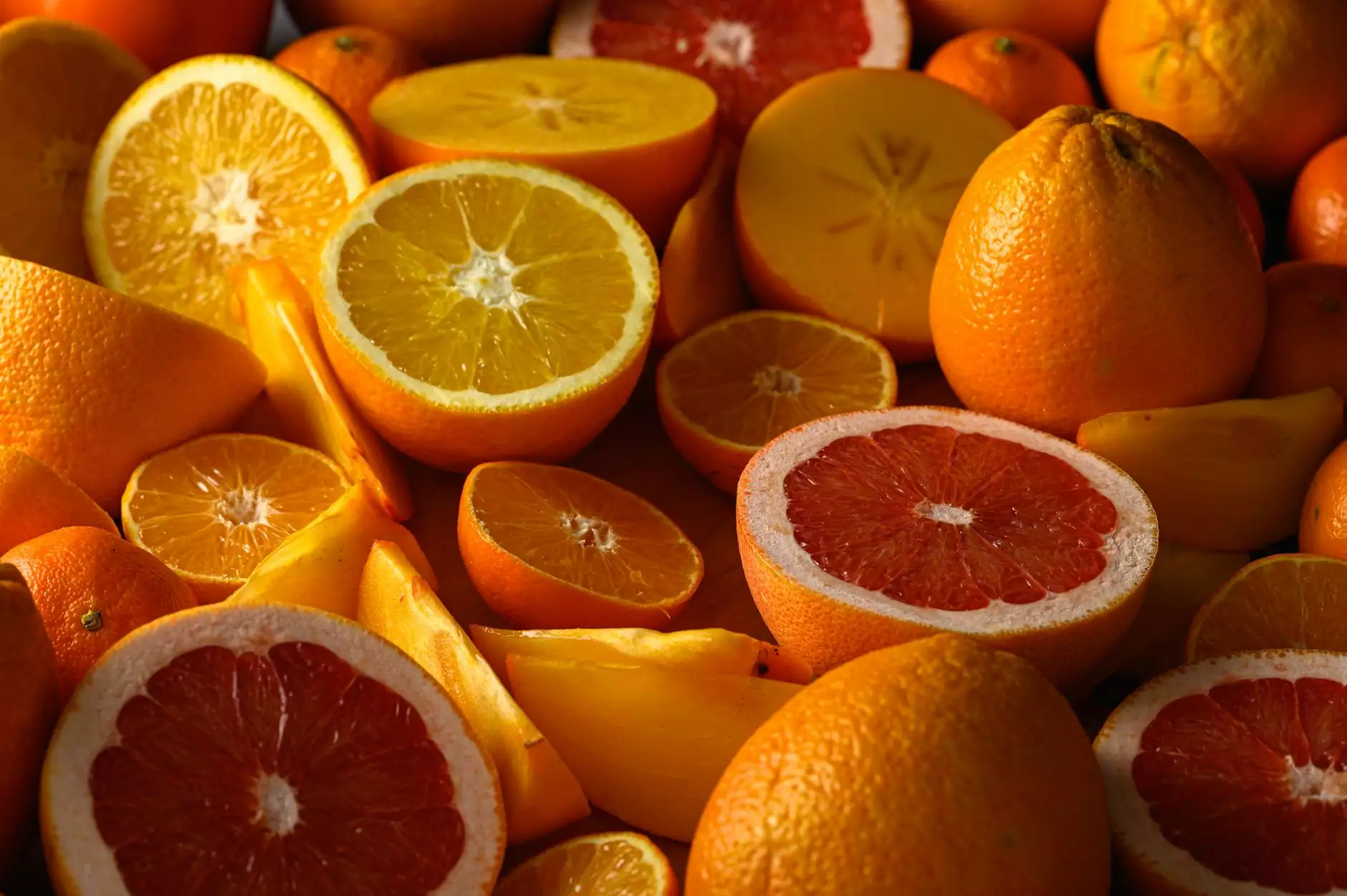
In today's digital landscape, the role of artificial intelligence (AI) and machine learning (ML) is paramount in driving business innovation and efficiency. Central to this technology revolution is the concept of semantic segmentation, a powerful technique that allows machines to understand visual data on a pixel-by-pixel basis. At the forefront of this transformational journey are semantic segmentation labeling tools that streamline the process of preparing data for machine learning models. In this article, we delve into why these tools are crucial for businesses, especially in the realm of software development, and how they empower teams to achieve greater accuracy and efficiency.
Understanding Semantic Segmentation
Semantic segmentation is a computer vision task that involves classifying each pixel in an image into categories. This allows a machine not only to identify objects within an image but also to understand the relationships and context around them. For example, in a self-driving car application, understanding the difference between a pedestrian, a vehicle, and the road surface is essential for safe navigation.
Why Is Semantic Segmentation Important?
The significance of semantic segmentation lies in its ability to enable machines to make more informed decisions based on the detailed analysis of visual data. Some key benefits include:
- Enhanced Object Recognition: Machines can recognize and differentiate between various objects with higher accuracy.
- Contextual Awareness: Machines can interpret the environment by analyzing how objects interact with each other.
- Improved User Experiences: Applications, especially in augmented reality (AR) and virtual reality (VR), become more intuitive and engaging.
What Are Semantic Segmentation Labeling Tools?
Semantic segmentation labeling tools are specialized software applications that assist users in annotating images with pixel-wise labels. These labels indicate which parts of an image belong to a specific category, hence preparing the data needed for training deep learning models.
Features of High-Quality Semantic Segmentation Labeling Tools
The effectiveness of a semantic segmentation labeling tool can often dictate the success of a machine learning project. A few key features to look for include:
- User-Friendly Interface: A straightforward interface allows users of all skill levels to navigate the tool effectively.
- Robust Annotation Capabilities: Tools should support polygon, box, and line annotations in addition to pixel-level segmentation.
- Collaboration Features: The ability for multiple users to work on projects simultaneously enhances productivity.
- Integration with ML Frameworks: Good tools should seamlessly export labeled data in formats that are compatible with popular ML frameworks.
The Business Case for Using Semantic Segmentation Labeling Tools
Investing in semantic segmentation labeling tools can yield substantial returns for businesses involved in software development. Some business advantages include:
1. Increased Efficiency
Automating the labeling process significantly reduces the time spent on data preparation. This allows machine learning engineers and developers to focus more on building and optimizing models rather than getting bogged down in data management.
2. Higher Quality Data
Accurate data is the backbone of successful machine learning projects. Tools that offer precision in labeling help minimize errors that could lead to inaccurate model predictions.
3. Cost-Effectiveness
By streamlining the annotation process, businesses can save on labor costs associated with manual labeling. Furthermore, more accurate data means fewer resources spent on retraining models due to faulty inputs.
Choosing the Right Semantic Segmentation Labeling Tool
With several options available in the market, selecting the right semantic segmentation labeling tool can be daunting. Here are some tips to consider during the selection process.
Assess Your Team's Needs
Consider the specific requirements of your business and your team’s expertise. Tools tailored for specific industries, such as healthcare or automotive, may provide additional functionality that can be beneficial.
Trial and Feedback
Many tools offer trial versions. Utilize these to gather feedback from team members who will be using the tool on a regular basis. Their insights can guide your choice.
Look for Community and Support
A robust community and dedicated support team can be invaluable. They not only help in troubleshooting but also often provide additional resources, tutorials, and best practices.
Case Studies: Success Stories Using Semantic Segmentation Labeling Tools
1. Autonomous Vehicles
Companies within the autonomous vehicle sector have leveraged semantic segmentation to train their models. By using semantic segmentation labeling tools, they can accurately annotate millions of driving scenes, enabling cars to better interpret complex environments.
2. Medical Imaging
In healthcare, semantic segmentation is used for analyzing medical images. Tools allow practitioners to label tumors or other abnormalities with precision, enhancing diagnostic accuracy and patient outcomes.
Future Trends in Semantic Segmentation and Labeling Tools
As machine learning technology continues to evolve, so too will the features of semantic segmentation labeling tools. Here are some trends to watch:
- AI-Assisted Labeling: Tools that utilize AI to assist in the labeling process will become increasingly common, allowing for quicker and more accurate annotations.
- Integration with Cloud Technologies: Expect more tools to offer cloud-based solutions for seamless collaboration across distributed teams.
- Enhanced Real-Time Collaboration: As remote work becomes more prevalent, features that support real-time collaboration will be critical.
Conclusion
In conclusion, the implementation of semantic segmentation labeling tools is a game-changer for businesses engaged in software development and AI. By harnessing the power of these tools, organizations can enhance their data preparation processes, improve model accuracy, and ultimately achieve superior business outcomes. As the demand for intricate visual analysis continues to grow, embracing these technologies will be essential in gaining a competitive edge. With solutions like those offered by Keymakr, businesses can navigate the evolving landscape of machine learning with confidence and innovation.