Unlocking the Potential of Semantic Segmentation Video for Enhanced Data Annotation
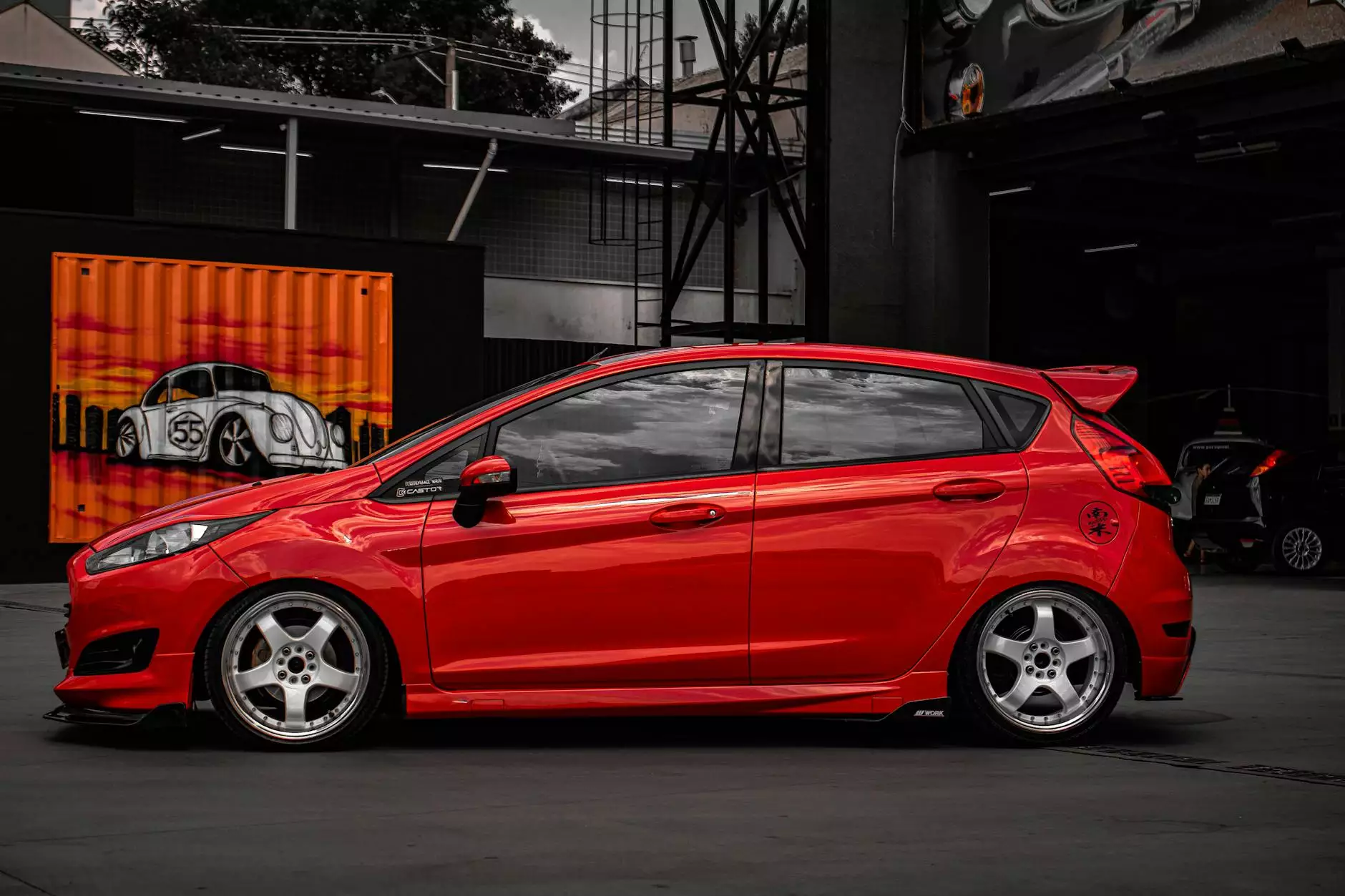
In the rapidly evolving landscape of artificial intelligence, the ability to accurately interpret visual information is paramount. One of the groundbreaking techniques used in AI today is semantic segmentation video. This powerful approach is at the core of many advanced applications, including autonomous vehicles, healthcare imaging, and augmented reality. By diving deep into the intricacies of semantic segmentation, we can better understand how businesses can leverage data annotation tools and platforms to enhance their AI capabilities.
What is Semantic Segmentation?
Semantic segmentation is a process where an algorithm categorizes each pixel of an image into a predefined class label, effectively enabling the machine to understand not just the objects within an image, but also their context. In the realm of video, this means that every frame of the video is analyzed, resulting in precise delineation of objects over time.
The Importance of Video Segmentation in AI
The significance of semantic segmentation video cannot be overstated. In various industries, the capacity to segment video data accurately allows for:
- Improved Object Recognition: The algorithm can distinguish between different objects, enhancing the accuracy of recognition tasks.
- Contextual Understanding: Understanding the relationships between objects in a scene, thereby improving decision-making processes.
- Real-time Processing: Essential for applications like surveillance, where quick responses are critical.
Application Scenarios of Semantic Segmentation Video
Let’s explore several industries where semantic segmentation video is making a significant impact:
1. Autonomous Vehicles
In the automotive industry, semantic segmentation is crucial for real-time navigation and obstacle avoidance. Vehicles equipped with camera systems can interpret their surroundings by identifying lanes, pedestrians, and other vehicles, enhancing safety and efficiency.
2. Healthcare
In medical imaging, segmenting video data helps in accurately detecting diseases. For instance, identifying cancerous cells in video endoscopies can lead to earlier diagnosis and better treatment plans.
3. Robotics
Robots often depend on accurate environmental data. With semantic segmentation, robots can navigate complex settings such as warehouses or homes, allowing them to interact safely with humans and perform tasks efficiently.
4. Augmented Reality (AR)
AR applications rely heavily on understanding the environment in real-time. Semantic segmentation allows AR systems to overlay virtual objects correctly onto the real world, providing an immersive experience.
Keylabs.ai: Pioneering Data Annotation Solutions
Keylabs.ai is at the forefront of providing sophisticated data annotation tools and platforms specifically designed to cater to the needs of businesses utilizing semantic segmentation. Our solutions include:
Data Annotation Tool
Our data annotation tool allows users to label their video datasets efficiently, streamlining the preparation of data necessary for training machine learning models. Features include:
- User-Friendly Interface: An intuitive design making it easy for annotators to navigate.
- Custom Annotation Classes: Users can define their own classes based on the needs of their project.
- Real-Time Collaboration: Multiple users can work simultaneously, enhancing productivity.
Data Annotation Platform
The comprehensive data annotation platform provided by Keylabs.ai is robust and highly scalable, suitable for enterprises of all sizes. Key features include:
- Scalability: Handle large volumes of data effortlessly.
- Quality Assurance: Quality control measures ensure high accuracy in annotations.
- Integration Capabilities: Seamlessly integrate with existing data pipelines.
Challenges in Semantic Segmentation
Despite its potential benefits, there are challenges in implementing semantic segmentation effectively:
- Data Quality: The accuracy of semantic segmentation heavily relies on the quality of the training data.
- Complexity of Scenes: Highly dynamic environments can complicate the segmentation process.
- Resource Intensity: High computational power is often required, which may be a barrier for smaller businesses.
Future Trends in Semantic Segmentation and Data Annotation
The field of semantic segmentation is constantly evolving. Here are some future trends to watch:
- Advancements in Deep Learning: Ongoing research in deep learning will likely lead to more sophisticated segmentation algorithms.
- Increased Automation: Tools are becoming more automated, requiring less manual intervention for data annotation.
- Cloud-Based Solutions: The shift towards cloud-based platforms will enable more accessible and scalable data annotation services.
Conclusion: Embracing Data Annotation for Business Growth
In conclusion, semantic segmentation video is a transformative technique that is crucial to the success of various industries focused on artificial intelligence. By adopting advanced data annotation tools and platforms like those offered by Keylabs.ai, businesses can enhance their data processing capabilities, leading to improved outcomes and increased competitiveness in the market.
As AI continues to integrate into various aspects of our lives, understanding and implementing techniques like semantic segmentation will be vital for any business looking to thrive in the digital age. Embrace these changes, invest in quality data annotation, and watch as your business flourishes.